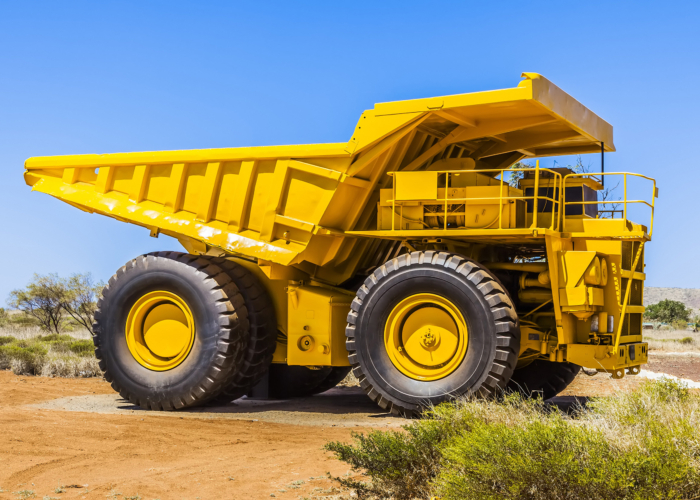
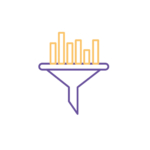
Background
Mining heavy machinery leasing company intended to enable the operators in the field to determine the root cause of equipment failures. Provided the right diagnosis, the great majority of failures could be rectified in the field without the need for equipment decommissioning leading to tremendous savings.
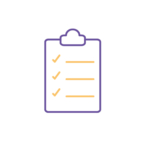
Objective
Develop a Machine Learning predictive model able to predict the root cause of failures. The equipment contains dozens of hydraulic pumps that are monitored by numerous sensors. The input to the predictive model is a subset of sensor readings.

Outcome
The predictive Machine Learning model (XGBoost) was able to correctly predict 98% of positives (Recall). Additionally, 54% of predicted positives were correct (Precision). This is a remarkable outcome given severely imbalanced training dataset.